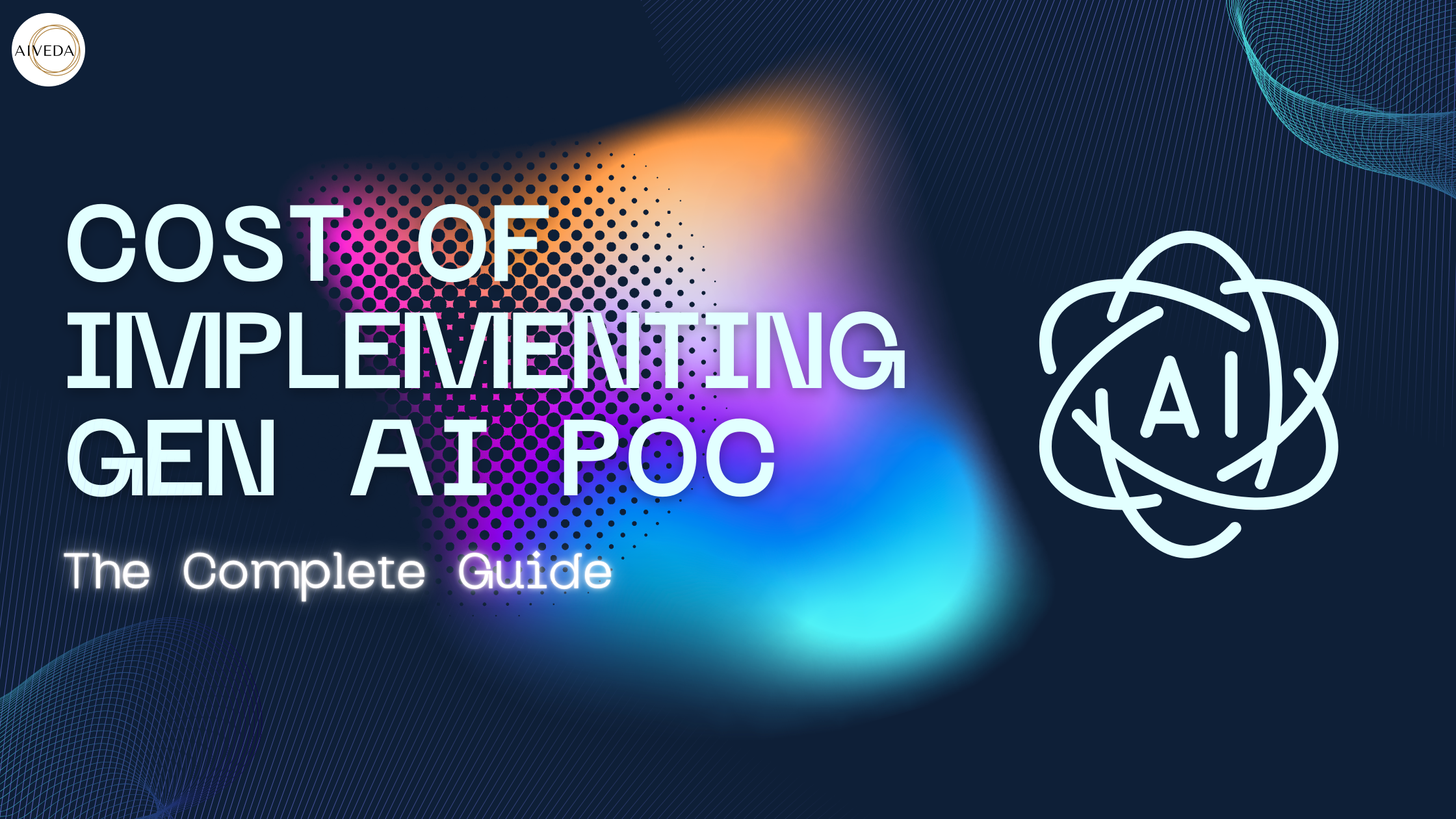
Generative AI (Gen AI) is rapidly transforming industries by enabling businesses to automate content creation, enhance customer experiences, and innovate faster than ever before. However, before fully committing to AI implementation, many enterprises start with a Generative AI Proof of Concept (POC) to assess feasibility, potential benefits, and costs. This blog will provide an in-depth look at the Gen AI POC cost, the factors that influence it, and how enterprises can strategically invest in Gen AI solutions.
What is a Generative AI POC?
A Generative AI Proof of Concept (Gen AI POC) is a preliminary project designed to test the viability and effectiveness of generative AI technologies within a specific business context. The POC allows enterprises to experiment with AI models, understand their capabilities, and evaluate their impact before scaling the solution across the organization.
Why Enterprises Should Consider a Gen AI POC
Implementing a Gen AI POC offers several advantages, including:
- Risk Mitigation: A POC allows businesses to test AI solutions in a controlled environment, reducing the risk of full-scale implementation.
- Cost Efficiency: By starting with a POC, enterprises can better estimate the total cost of deploying Gen AI solutions across their operations.
- Faster Innovation: A POC accelerates innovation by providing rapid insights into the feasibility and effectiveness of AI solutions.
- Informed Decision-Making: The results of a POC provide valuable data that help enterprises make informed decisions about investing in AI technologies.
Key Components of Gen AI Solutions
Before diving into the Gen AI POC cost, it’s essential to understand the core components that make up generative AI solutions:
1. AI Model Development
The development of AI models is at the heart of Gen AI solutions. Depending on the complexity of the task (e.g., text generation, image creation, video synthesis), different models such as GPT, GANs, or other neural networks may be used. Developing these models requires significant expertise in machine learning and deep learning.
2. Data Preparation
Data is the backbone of AI, and high-quality datasets are crucial for training effective generative models. Data preparation involves collecting, cleaning, and labeling data to ensure it is suitable for training AI models. The quality and quantity of data directly impact the performance of the AI model.
3. Training and Tuning
Training an AI model involves feeding it with large datasets and allowing it to learn patterns, structures, and relationships within the data. Tuning the model is an iterative process where parameters are adjusted to improve accuracy and performance. This stage is resource-intensive and often constitutes a significant portion of the Gen AI POC cost.
4. Infrastructure and Cloud Services
Generative AI models require powerful computational resources, especially during the training phase. Many enterprises rely on cloud services like Google Cloud, AWS, or Azure to provide the necessary GPU/TPU power. The cost of cloud services is a key factor in the overall POC budget.
5. Integration and Testing
Once the AI model is trained, it must be integrated into existing systems and workflows. Testing ensures that the AI solution functions correctly and meets the desired outcomes. This phase may also involve the development of user interfaces and APIs to interact with the AI system.
Understanding Gen AI POC Cost
The cost of a Gen AI POC can vary widely based on several factors, including the complexity of the AI model, the scope of the project, and the resources required. Below is a detailed breakdown of the key cost drivers:
1. AI Model Development Costs
The cost of developing a generative AI model depends on the complexity of the model and the expertise required. Simple models that generate basic text or images might cost between $20,000 to $50,000, while more advanced models that require complex neural networks can cost upwards of $100,000.
Factors Influencing AI Model Development Costs:
- Model Complexity: Advanced models like GPT-3 or GANs require more development time and resources.
- Expertise: Hiring skilled data scientists and AI developers increases costs.
- Licensing: Some AI tools and platforms require licensing fees, adding to the development cost.
2. Data Preparation Costs
High-quality data is essential for training effective AI models, and the process of data preparation can be costly. Depending on the data’s complexity and volume, data preparation costs can range from $10,000 to $30,000.
Factors Influencing Data Preparation Costs:
- Data Volume: Larger datasets require more time and resources to process.
- Data Complexity: Unstructured or complex data types, such as video or audio, increase costs.
- Data Quality: Ensuring data accuracy and consistency may involve additional cleaning and labeling efforts.
3. Training and Tuning Costs
Training a generative AI model is one of the most resource-intensive stages, often accounting for a significant portion of the Gen AI POC cost. Depending on the model’s complexity and the computational resources required, training costs can range from $15,000 to $50,000.
Factors Influencing Training Costs:
- Compute Power: The need for high-performance GPUs/TPUs increases cloud service costs.
- Training Duration: Longer training times result in higher expenses.
- Tuning Efforts: Fine-tuning the model to achieve optimal performance may require multiple iterations, adding to the cost.
4. Infrastructure and Cloud Service Costs
Leveraging cloud infrastructure to train and deploy generative AI models provides flexibility and scalability, but it also comes with ongoing costs. Cloud service costs can range from $10,000 to $40,000 for a POC, depending on the duration and intensity of usage.
Factors Influencing Cloud Service Costs:
- Compute Requirements: Models with high computational demands incur higher cloud usage costs.
- Storage Needs: Storing large datasets and model versions can increase costs.
- Service Level: Premium support and advanced cloud features may come at an additional cost.
5. Integration and Testing Costs
Integrating the AI model with existing systems and performing rigorous testing is crucial for ensuring the POC’s success. Integration and testing costs can range from $10,000 to $25,000.
Factors Influencing Integration and Testing Costs:
- System Complexity: Integrating with multiple systems or platforms increases complexity and costs.
- User Interface Development: Creating user-friendly interfaces to interact with the AI model adds to the cost.
- Testing Scope: Comprehensive testing across different scenarios and environments may require more resources.
Total Gen AI POC Cost Estimation
Based on the factors discussed above, a rough estimate of the Gen AI POC cost could range from $65,000 to $200,000, depending on the project’s scope, complexity, and specific requirements. Here’s a summary breakdown:
- AI Model Development: $20,000 – $100,000
- Data Preparation: $10,000 – $30,000
- Training and Tuning: $15,000 – $50,000
- Infrastructure and Cloud Services: $10,000 – $40,000
- Integration and Testing: $10,000 – $25,000
How to Optimize Gen AI POC Cost
While the costs associated with a Gen AI POC can be significant, there are several strategies enterprises can use to optimize their investment:
1. Start with a Clear Objective
Define clear, specific objectives for the POC to ensure that resources are focused on achieving tangible results. This helps avoid unnecessary spending on features or capabilities that do not align with the project’s goals.
2. Leverage Existing Data
Use existing data where possible to reduce data preparation costs. If you have high-quality data available, you can save time and resources on data collection and cleaning.
3. Choose the Right Cloud Provider
Select a cloud provider that offers the best balance of cost and performance for your needs. Consider using spot instances or reserved instances to reduce compute costs.
4. Iterative Development
Adopt an iterative development approach, where you start with a minimal viable product (MVP) and gradually add features based on feedback and results. This reduces the risk of over-investing in features that may not be necessary.
5. Optimize Model Training
Optimize the training process by using techniques such as transfer learning, which leverages pre-trained models to reduce the amount of training data and computational power required.
Conclusion: Budgeting for Gen AI Solutions
Investing in a Gen AI POC is a strategic step towards leveraging AI to drive innovation and efficiency within your enterprise. By understanding the key cost drivers and following best practices, you can optimize your POC investment and set the stage for successful AI implementation.
When planning your Gen AI POC cost, it’s essential to work with experienced AI developers and data scientists who can guide you through the process, helping you achieve your objectives while staying within budget. With careful planning and execution, a Generative AI POC can deliver valuable insights and pave the way for full-scale AI adoption.
Ready to Explore Gen AI Solutions? Contact us to learn how we can help you design and implement a cost-effective Generative AI POC tailored to your business needs.