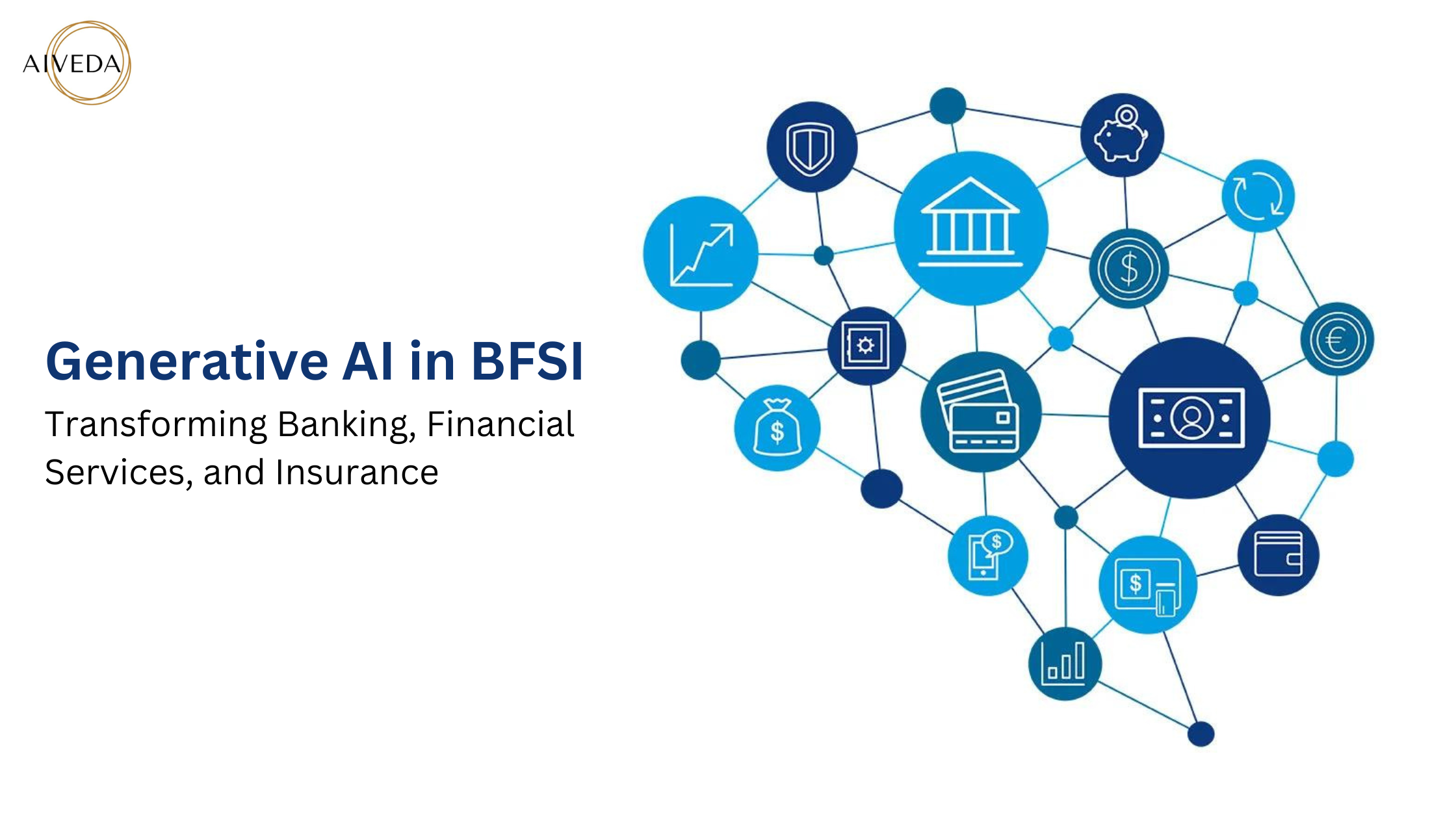
Generative AI, an advanced subset of artificial intelligence, is transforming industries worldwide. In the banking, financial services, and insurance (BFSI) sector, the impact of Generative AI is particularly profound. This blog delves into the technical aspects, applications, and benefits of Generative AI in BFSI, illustrating how it is reshaping the industry.
Understanding Generative AI in BFSI
- Definition and Core Concepts
Generative AI involves creating new data that mimics a given dataset. Unlike traditional AI, which focuses on pattern recognition and prediction, Generative AI generates new content. This capability is crucial in BFSI for tasks such as fraud detection, personalized customer service, and financial forecasting.
- Underlying Architectures
Generative AI primarily utilizes two architectures: Generative Adversarial Networks (GANs) and Transformer models. GANs consist of two neural networks—the generator and the discriminator—that work in tandem to create realistic data. Transformer models, like GPT-4, use self-attention mechanisms to process and generate sequences of data, making them powerful tools for language-based tasks.
- Training Processes
Generative AI models undergo extensive training using supervised, unsupervised, and reinforcement learning. This training requires large datasets and significant computational resources, often leveraging high-performance GPUs and cloud platforms to manage the demands.
Applications of Generative AI in BFSI
1. Fraud Detection and Prevention
Generative AI enhances fraud detection by creating synthetic fraud scenarios to train models. These scenarios help identify unusual patterns and detect fraudulent activities more accurately.
Case Study: Using GANs for Fraud Detection
Banks use GANs to simulate fraudulent transactions, which are then used to train detection models. This approach improves the accuracy and speed of identifying fraud, reducing financial losses.
Technical Aspects
- Data Collection: Gathering transaction data, including normal and fraudulent transactions.
- Model Training: Using GANs to create synthetic data and training the detection model.
- Deployment: Integrating the trained model into the bank’s transaction monitoring system.
2. Personalized Customer Service
Generative AI powers chatbots and virtual assistants that provide personalized customer service. These AI-driven systems handle customer inquiries, offer financial advice, and streamline customer support.
Example Implementation: Chatbots in Banking
Banks implement AI chatbots using Transformer models like GPT-4 to manage customer queries. These chatbots understand and respond to customer needs, providing 24/7 support.
Technical Challenges
- Natural Language Understanding: Ensuring the chatbot comprehends complex customer queries.
- Context Maintenance: Maintaining context over long interactions to provide relevant responses.
- Data Privacy: Protecting sensitive customer information during interactions.
3. Financial Forecasting and Risk Management
Generative AI models analyze historical financial data to predict future trends and manage risks. These predictions help financial institutions make informed decisions.
Predictive Analytics with LSTM Networks
Long Short-Term Memory (LSTM) networks, a type of recurrent neural network, are used to predict financial market trends and assess risks. This capability enables better investment strategies and risk mitigation.
Technical Implementation
- Data Preprocessing: Cleaning and normalizing historical financial data.
- Model Training: Training LSTM networks on processed data to learn patterns.
- Forecasting: Using trained models to predict future market trends and risks.
4. Automated Document Processing
Generative AI automates the processing of financial documents, such as loan applications and insurance claims. This automation speeds up workflows and reduces human error.
Example: Using GPT-4 for Document Analysis
Financial institutions use GPT-4 to analyze and extract relevant information from documents, automating the approval process and improving efficiency.
Technical Details
- Data Extraction: Identifying and extracting key information from documents.
- Model Training: Training models on a variety of document types to ensure accuracy.
- Integration: Deploying the model within the institution’s document management system.
Benefits of Generative AI in BFSI
Operational Efficiency and Cost Reduction
Generative AI automates repetitive tasks, leading to significant cost savings and operational efficiency. Financial institutions can allocate resources more strategically, focusing on high-value activities.
Improved Customer Satisfaction and Loyalty
Personalized services and faster response times enhance customer satisfaction. Metrics such as Net Promoter Score (NPS) and Customer Satisfaction (CSAT) reflect improved customer experiences resulting from AI-driven services.
Enhanced Risk Management
Generative AI’s predictive capabilities improve risk assessment and management. Financial institutions can better anticipate market trends and mitigate risks, ensuring financial stability.
Data-Driven Decision Making
Real-time analytics powered by Generative AI provide actionable insights, facilitating informed decision-making and strategic planning.
Challenges and Considerations
Data Privacy and Ethical Implications
Compliance with regulations such as GDPR and CCPA is crucial for protecting customer data. Ethical considerations involve ensuring fairness and addressing biases in AI-generated content.
Technical Considerations
Model interpretability and explainability are essential for understanding AI decisions. Robustness ensures models perform reliably across different scenarios.
Integration with Existing IT Infrastructure
Seamlessly integrating AI systems with existing IT infrastructure requires careful planning and execution.
Balancing Automation with Human Oversight
Designing hybrid human-AI workflows ensures that while automation handles routine tasks, human oversight is available for complex issues, maintaining a balance between efficiency and empathy.
Future Trends in Generative AI for BFSI
Evolution of AI Technologies
Advancements in neural architecture search (NAS) and automated machine learning (AutoML) will revolutionize AI capabilities, making it easier to develop and deploy powerful models.
Emerging Use Cases
Real-time sentiment analysis, voice-enabled AI assistants, and augmented reality (AR) applications represent exciting new frontiers for Generative AI in enhancing customer experiences.
Long-Term Vision
The convergence of Generative AI with IoT and edge computing will create more interactive and responsive customer experiences. This integration will enable real-time data processing and personalized interactions on a massive scale.
Research Frontiers
Exploring unsupervised and self-supervised learning methods promises to improve the quality and efficiency of generative models, making them more adaptable and robust.
Conclusion
Generative AI is transforming the BFSI sector by enhancing fraud detection, personalizing customer service, improving financial forecasting, and automating document processing. By leveraging advanced technologies and innovative applications, financial institutions can improve operational efficiency, customer satisfaction, and risk management. As AI technologies continue to evolve, their impact on BFSI will only grow, making Generative AI an essential tool for staying competitive in the digital age. Embrace Generative AI to unlock its full potential and drive success in the BFSI sector.