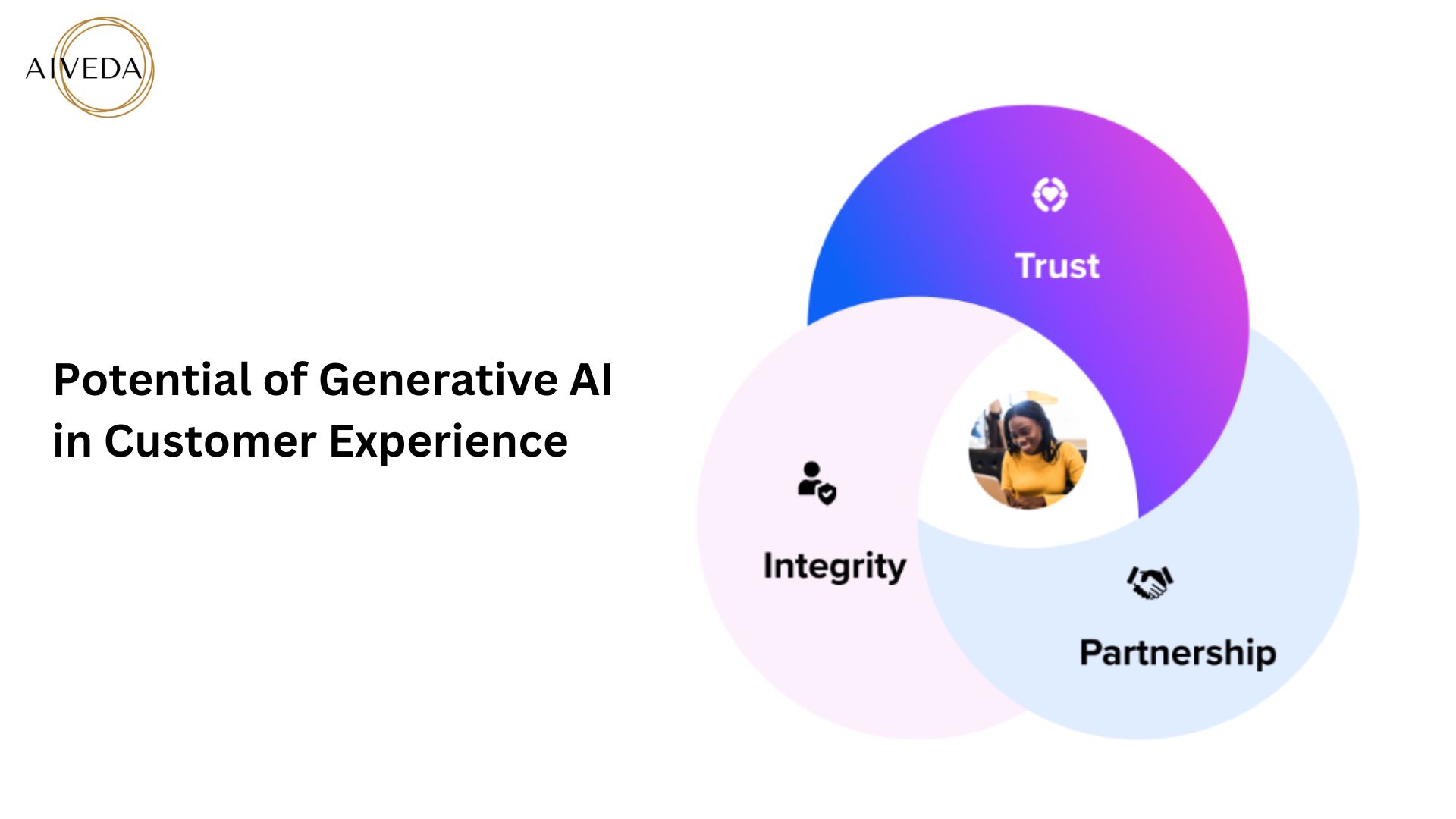
Generative AI is reshaping the landscape of artificial intelligence research and development. This innovative technology has profound implications for various sectors, including customer experience (CX), where its impact is becoming increasingly evident. As businesses strive to differentiate themselves in competitive markets, customer experience has emerged as a critical factor for success. Generative AI models, such as GPT-4, are disrupting traditional CX paradigms, offering unprecedented personalization, automation, and insights.
Understanding Generative AI
Definition and Core Concepts
Generative AI refers to artificial intelligence systems designed to generate new content, such as text, images, or music, based on the data they have been trained on. Unlike traditional AI, which relies on recognizing patterns in existing data, generative AI creates new data, making it highly versatile for various applications.
Underlying Architectures: GANs and Transformers
Generative AI primarily uses two types of architectures: Generative Adversarial Networks (GANs) and Transformer models. GANs consist of two neural networks, the generator and the discriminator, which work together to create realistic data. Transformers, like GPT-3 and GPT-4, leverage self-attention mechanisms to process and generate sequences of data, making them exceptionally powerful for tasks such as natural language processing.
Training Processes
Generative AI models are trained using supervised learning, unsupervised learning, and reinforcement learning. Supervised learning involves training the model on labeled data, while unsupervised learning allows the model to find patterns in unlabeled data. Reinforcement learning uses a reward-based system to train the model through trial and error.
Technical Overview of Model Training
Training generative AI models requires extensive datasets and significant computational resources. High-performance GPUs and cloud computing platforms are often used to handle the computational demands. The training process involves data preprocessing, model tuning, and iterative refinement to achieve high accuracy and performance.
Advanced Applications of Generative AI in Customer Experience
1. Personalized Customer Interactions
- Algorithmic Personalization
Generative AI enhances algorithmic personalization through advanced recommendation systems. These systems use collaborative filtering, content-based filtering, and hybrid models to tailor experiences to individual preferences.
- Case Study: Dynamic Content Generation with GPT-4
One practical application is using GPT-4 for personalized marketing emails. By analyzing user behavior and preferences, GPT-4 generates customized content that resonates with recipients, improving engagement rates and conversion.
- Technical Aspects of Real-Time Interface Customization
Real-time customization involves predictive analytics and user behavior modeling. Generative AI models analyze interactions and predict user needs, enabling dynamic adjustments to interfaces, enhancing user satisfaction.
2. Customer Support Automation
- Architecture of AI-Driven Chatbots
Generative AI powers advanced chatbots capable of intent recognition, entity extraction, and dialog management. These chatbots use Natural Language Processing (NLP) and Natural Language Understanding (NLU) to comprehend and respond to user queries effectively.
- Example Implementation: Using BERT and GPT-3
BERT can be used for intent classification, determining what the user wants to achieve, while GPT-3 generates appropriate responses, creating a seamless and natural interaction.
- Technical Challenges
Developing these systems involves challenges such as maintaining context over long conversations, handling ambiguous queries, and ensuring responses feel human-like. Overcoming these challenges requires sophisticated algorithms and extensive training.
3. Enhanced Customer Insights
- Data Preprocessing Techniques
Effective customer data analysis starts with data cleaning, normalization, and feature engineering. These steps ensure that the data used is accurate and relevant, improving the quality of insights derived.
- Leveraging Deep Learning Models
Deep learning models, including clustering algorithms and neural networks, segment customers into meaningful groups. This segmentation helps in understanding customer needs and tailoring services accordingly.
- Predictive Analytics
Implementing Long Short-Term Memory (LSTM) networks for predicting customer churn and estimating customer lifetime value (CLV) provides actionable insights for retention strategies and personalized marketing.
- Automated Report Generation
Transformers can be used for generating comprehensive reports automatically, which are then deployed in business intelligence platforms. These reports offer valuable insights and support data-driven decision-making.
4. Content Creation for Engagement
- Automated Content Generation Pipelines
Generative AI can automate content creation by collecting relevant data, designing content templates, and fine-tuning models to produce engaging material tailored to target audiences.
- Technical Deep Dive: Using GANs for Image Generation
GANs can create realistic images for personalized marketing campaigns, enhancing visual appeal and engagement.
- Dynamic Storytelling with AI
Sequence-to-sequence models generate coherent and contextually relevant narratives, providing dynamic storytelling capabilities that captivate audiences.
- Performance Metrics
Evaluating AI-generated content involves metrics like perplexity and BLEU scores to ensure quality and relevance.
Benefits of Generative AI in CX
- Operational Efficiency and Cost Reduction
Generative AI streamlines operations by automating routine tasks, leading to significant cost savings. This efficiency enables businesses to allocate resources more strategically.
- Improved Customer Satisfaction and Loyalty
Metrics such as Net Promoter Score (NPS) and Customer Satisfaction (CSAT) indicate improved customer experiences and loyalty resulting from personalized interactions and efficient support.
- Scalability Challenges and Solutions
Deploying AI systems for large-scale personalization poses scalability challenges. Solutions include optimizing algorithms and using distributed computing to handle increased loads.
- Data-Driven Decision Making
Real-time analytics dashboards integrated with AI systems provide actionable insights, facilitating informed decision-making and strategic planning.
Challenges and Considerations
- Data Privacy and Ethical Implications
Ensuring compliance with regulations like GDPR and CCPA is critical for protecting customer data and maintaining trust. Ethical considerations also involve addressing biases in AI-generated content to ensure fairness.
- Technical Considerations
Model interpretability and explainability are essential for understanding AI decisions. Robustness ensures models perform reliably across different scenarios.
- Integration with Existing IT Infrastructure
Seamlessly integrating AI systems with existing IT infrastructure and CRM systems requires careful planning and execution.
- Balancing Automation with Human Oversight
Designing hybrid human-AI workflows ensures that while automation handles routine tasks, human oversight is available for complex issues, maintaining a balance between efficiency and empathy.
Future Trends in Generative AI for CX
- Evolution of AI Technologies
Advancements in neural architecture search (NAS) and automated machine learning (AutoML) are set to revolutionize AI capabilities, making it easier to develop and deploy powerful models.
- Emerging Use Cases
Real-time sentiment analysis, voice-enabled AI assistants, and augmented reality (AR) applications represent exciting new frontiers for Generative AI in enhancing customer experiences.
- Long-Term Vision
The convergence of Generative AI with IoT and edge computing will create more interactive and responsive customer experiences. This integration will enable real-time data processing and personalized interactions on a massive scale.
- Research Frontiers
Exploring unsupervised and self-supervised learning methods promises to improve the quality and efficiency of generative models, making them more adaptable and robust.
Leveraging Generative AI for Market Research
- Enhanced Data Collection and Analysis
Generative AI facilitates comprehensive market research by automating data collection from various sources, including social media, surveys, and customer feedback. This automation enables businesses to gather extensive and diverse data sets, providing a more comprehensive understanding of market trends and consumer preferences.
- Predictive Market Trends
Using generative AI, businesses can predict market trends by analyzing historical data and identifying patterns. These predictions help companies stay ahead of market changes and adapt their strategies accordingly.
- Customer Sentiment Analysis
Generative AI models analyze customer sentiments by processing text data from reviews, social media, and feedback forms. This analysis provides insights into customer opinions and experiences, helping businesses improve their products and services.
Implementing Generative AI in Sales Strategies
- Personalized Sales Pitches
Generative AI creates personalized sales pitches by analyzing customer data and identifying individual preferences. This personalization increases the likelihood of conversion by addressing the specific needs and interests of each customer.
- Sales Forecasting
Generative AI enhances sales forecasting by analyzing historical sales data and predicting future trends. Accurate forecasting helps businesses manage inventory, plan marketing campaigns, and set realistic sales targets.
- Lead Scoring and Nurturing
Generative AI models assess and score leads based on their likelihood to convert. This scoring helps sales teams prioritize their efforts and nurture high-potential leads with tailored content and offers.
Enhancing Employee Training and Development with Generative AI
- Personalized Training Programs
Generative AI creates personalized training programs by analyzing employee performance data and identifying skill gaps. Customized training ensures that employees receive relevant and effective learning experiences.
- Simulated Training Environments
Generative AI models create realistic simulations for training purposes, allowing employees to practice skills in a controlled environment. These simulations enhance learning outcomes and prepare employees for real-world scenarios.
- Performance Monitoring and Feedback
Generative AI monitors employee performance and provides real-time feedback, helping employees improve their skills and achieve their career goals. Continuous feedback fosters a culture of learning and development.
Conclusion
In conclusion, Generative AI holds transformative potential for reshaping customer experience landscapes. By leveraging advanced technologies and innovative applications, businesses can enhance personalization, automate support, gain deeper insights, and create engaging content. The strategic adoption of Generative AI, supported by best practices and ethical considerations, will drive significant improvements in customer satisfaction and operational efficiency. As AI technologies continue to evolve, their impact on customer experience will only grow, making Generative AI an essential tool for businesses looking to stay competitive in the digital age.