The Impact of Generative AI on Predictive Analytics
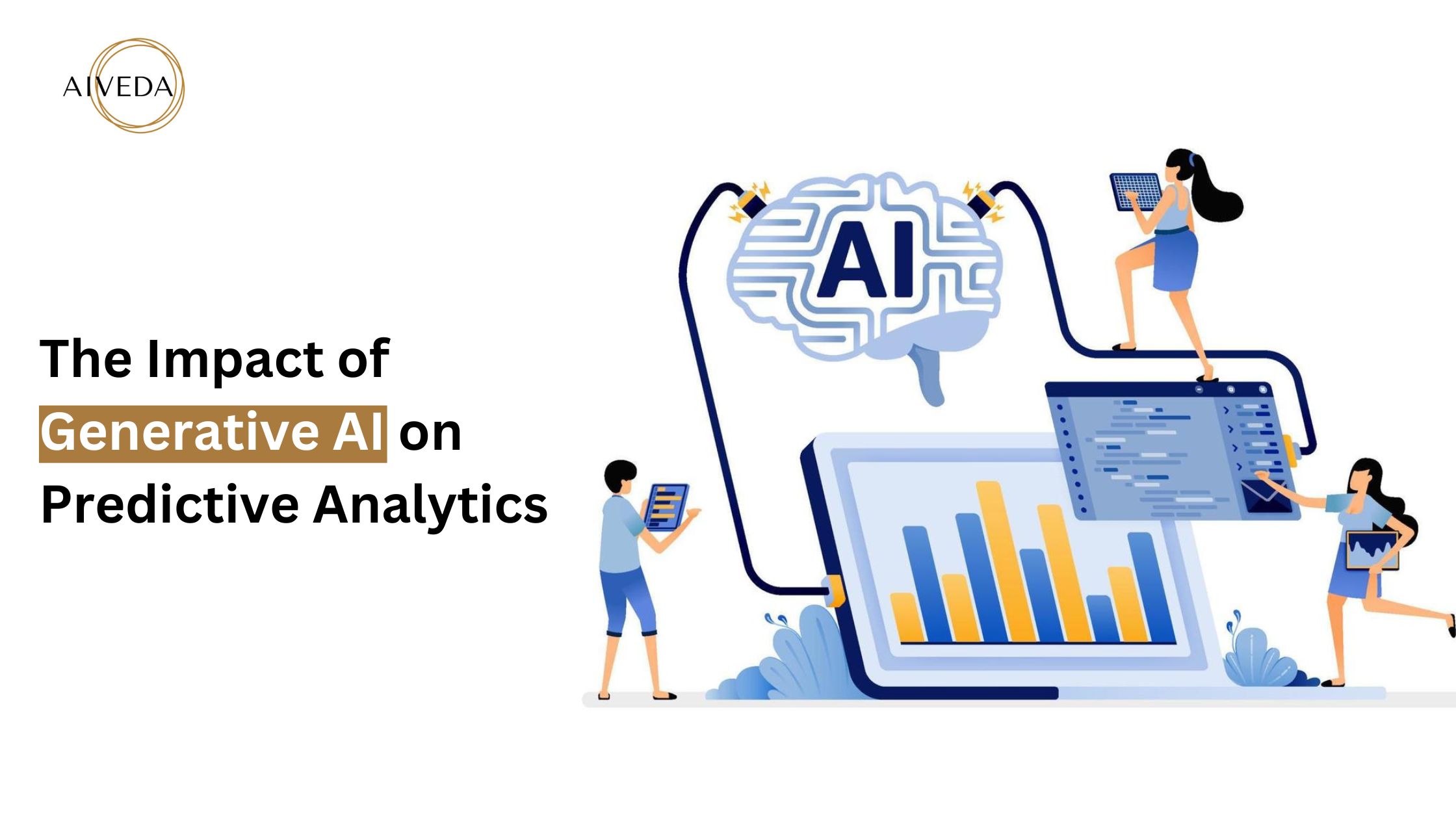
Introduction
Predictive analytics has been the compass guiding businesses through the murky waters of data-driven decision-making. It’s where patterns are deciphered, and the future is forecasted. Yet, the advent of Generative AI promises to not just navigate these waters but to chart new territories. This transformative technology is redefining the boundaries of data analysis, prediction accuracy, and decision-making precision. In this exploration, we delve into how Generative AI is reshaping the landscape of predictive analytics.
Understanding Predictive Analytics
Predictive analytics has been the cornerstone of strategic decision-making across a multitude of sectors. In healthcare, it forecasts patient outcomes, leading to better preventive care strategies. In finance, it predicts market movements, aiding investors in making more informed decisions. At its core, predictive analytics sifts through historical data to identify trends, patterns, and correlations that can offer a window into what the future might hold.
This analytical process combines various statistical techniques, including data mining, modeling, machine learning, and AI, to process and analyze large datasets. Businesses then use this analysis to predict future trends, behaviors, and events. The accuracy of these predictions can mean the difference between a profitable quarter and a significant loss, a successful product launch, or a recall.
However, predictive analytics is not a panacea. It grapples with challenges such as data scarcity, where the lack of sufficient data points can render predictive models less reliable or even unusable. Data quality is another concern; inaccurate or incomplete data sets can lead to predictions that are off the mark. Relevance is also critical — data that is not contextually relevant to the problem at hand can skew results, leading to flawed strategies.
Despite these hurdles, the value of predictive analytics is indisputable. It enables organizations to make proactive decisions, anticipate needs, and offer solutions before challenges escalate into problems. The next step is to overcome its limitations, which is where Generative AI begins to play a pivotal role.
Generative AI: The New Frontier
Generative AI marks a paradigm shift in our approach to data and analytics. It brings to the table an ability to generate new data – data that, while synthetic, is incredibly realistic and useful for training predictive models. At the heart of this capability are Generative Adversarial Networks (GANs), a class of machine learning frameworks designed by Ian Goodfellow and his colleagues in 2014. GANs pit two neural networks against each other: a generator that creates data and a discriminator that evaluates its authenticity.
The generator creates data instances that are indistinguishable from real data, while the discriminator assesses whether the data is real or synthetic. Through their rivalry, the generator learns to produce increasingly authentic data. This process continues until the discriminator can no longer differentiate synthetic data from actual data.
GANs and similar Generative AI technologies have revolutionized the scope of what’s possible in predictive analytics. By generating high-fidelity data, they provide a means to enhance datasets, improve model training, and ultimately, enable more accurate predictions. This is particularly beneficial in scenarios where data is sensitive or scarce, such as in medical trials or rare event prediction.
Generative AI is not limited to creating data that doesn’t exist; it also augments existing datasets, filling in gaps and expanding the breadth of data available for analysis. In doing so, it addresses the three primary challenges of predictive analytics: scarcity, quality, and relevance of data.
Enhancing Data Quality and Quantity
The alchemic capabilities of Generative AI in predictive analytics are nothing short of revolutionary. Synthetic data creation is not merely about quantity but quality. The generated data is not a crude facsimile but a refined product that can pass the stringent tests of authenticity. This serves as a powerful tool in scenarios where acquiring real-world data is difficult, costly, or impractical.
For instance, in financial markets, where real-time data is the lifeblood of trading algorithms, Generative AI can create additional data points to simulate market conditions. This synthetic data enables the testing of predictive models under a wide array of market scenarios, including those that are hypothetical or have not yet occurred.
Similarly, in healthcare, where patient privacy is paramount, Generative AI can create anonymized datasets that retain the statistical properties of the original data. Researchers and healthcare professionals can use these datasets without compromising patient confidentiality to develop predictive models for disease progression, treatment outcomes, and public health responses.
The quality of synthetic data also means that models trained on this data can capture a level of complexity and nuance previously unattainable. This translates into more accurate, reliable, and robust predictive analytics. Additionally, the diversity of data that Generative AI can produce ensures that the predictive models are not just accurate for a narrow range of scenarios but are generalizable across various conditions and populations.
The resulting enhancement in the quality and quantity of data available for predictive analytics is transformative. It allows for the creation of more nuanced and sophisticated models, which can predict outcomes with a higher degree of accuracy. This leap in predictive capability has the potential to impact every industry that relies on forecasting, from stock market predictions to anticipating geopolitical events.
Speed and Efficiency in Analytics
In the realm of predictive analytics, speed can be as valuable as accuracy. Generative AI accelerates the pace of data analysis, enabling businesses to iterate models and respond to emerging trends with alacrity. This section will explore the efficiency gains afforded by Generative AI and how they translate into competitive advantages.
Case Studies: Generative AI in Action
From virtual patient data in healthcare enabling better drug discovery to customer behavior simulations in retail, Generative AI is already making waves. This section will showcase several case studies where Generative AI’s impact on predictive analytics is not just theoretical but practical and measurable.
Ethical Considerations and Challenges
With great power comes great responsibility, and Generative AI is no exception. The ethical implications of synthetic data, privacy concerns, and the biases inherent in AI models are issues that must be tackled. This section will ponder the ethical quandary and the challenges that come with the territory of advanced AI.
The Future of Predictive Analytics with Generative AI
Looking to the horizon, Generative AI is set to be the guiding star for predictive analytics. This final section will speculate on future advancements in Generative AI and consider how these might further refine predictive models. It will also discuss the evolving skill set that data scientists will require to navigate this new landscape.
Conclusion
Generative AI is not just altering the predictive analytics game; it’s changing the playing field. As we conclude, we reflect on the profound impact of this technology and the potential it holds for businesses. The future is bright, and those who adapt to these new tools will find themselves leading the charge into a new era of analytics-driven strategy.