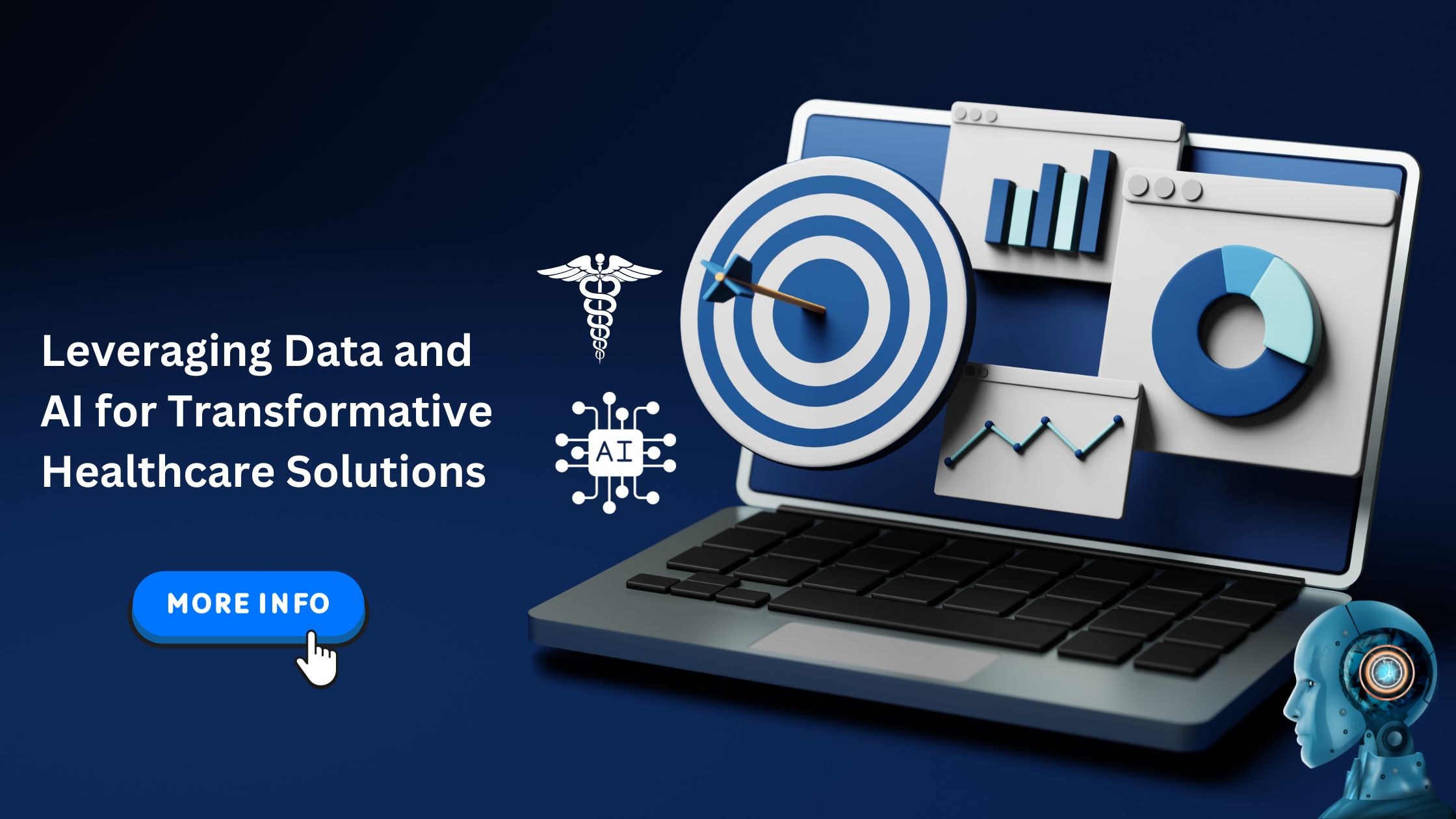
Introduction to Data and AI in Healthcare
The healthcare industry is changing quickly because of new technologies like Data Science and Artificial Intelligence (AI). These tools are becoming important parts of healthcare around the world. They offer new ways to improve how patients are treated, make healthcare operations more efficient, and personalize healthcare services.
The Amount of Data in Healthcare
Healthcare creates a lot of data. Electronic Health Records (EHRs), medical images, lab tests, and data from wearable devices all contribute to this. The challenge is not just the amount of data but also the different types and how quickly it’s created. Traditional ways of managing data can’t handle this, which leads to problems like data being stored in different places and not used effectively.
How AI is Changing Things
AI, especially Machine Learning and Deep Learning, can help make sense of all this data. These technologies can look through large and complex sets of data to find useful information. For example, AI can help predict if a patient will need to come back to the hospital, help doctors understand medical images, or help choose the best treatment plans.
Ethical Questions
Using Data and AI in healthcare also brings up ethical questions. These include concerns about keeping data private and secure, and making sure patients agree to how their data is used. Laws like HIPAA in the United States and GDPR in Europe are trying to address these issues, but the rules are still being developed.
Making Systems Work Together
Another important issue is making sure different healthcare systems can work together. As healthcare providers try to work in a more connected way, it’s important that different systems can share data. AI can help make this happen, which improves the overall quality of healthcare.
Customized Medicine
One of the most exciting uses of Data and AI in healthcare is customized medicine. By looking at a patient’s genetic information, lifestyle, and other factors, AI can suggest treatment plans that are specifically designed for each person. This can make treatments more effective and reduce side effects.
Saving Money
Using AI can also save money. A report by Accenture said that AI could save up to $150 billion a year for healthcare in the United States by 2026. These savings come from using automation, predicting future events, and preventing health issues before they happen.
Section 1: Data Security and Privacy in Healthcare
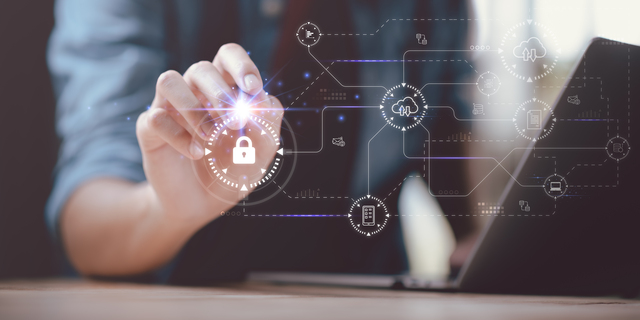
The Importance of Data Security
Data security is a critical concern in healthcare. With the increasing use of Electronic Health Records (EHRs), wearable devices, and telemedicine, the amount of digital data generated is enormous. This data is a treasure trove of sensitive information, including patient histories, financial data, and other personal details. Unauthorized access to this data can lead to identity theft, financial fraud, and even medical errors.
Types of Threats
The healthcare industry faces a variety of threats when it comes to data security. These range from external threats like hacking and phishing attacks to internal threats such as accidental data leaks by employees. Ransomware attacks, where hackers encrypt data and demand a ransom for its release, have also targeted healthcare institutions.
Regulatory Compliance
Various laws and regulations govern the security of healthcare data. In the United States, the Health Insurance Portability and Accountability Act (HIPAA) sets the standard for protecting sensitive patient data. Similarly, the General Data Protection Regulation (GDPR) in Europe mandates stringent data protection requirements. Non-compliance can result in hefty fines and legal repercussions.
Role of AI in Data Security
AI can play a significant role in enhancing data security. Machine learning algorithms can monitor network behavior, detect anomalies, and flag potential security threats in real-time. AI can also automate the process of compliance checks, ensuring that the healthcare institution is always in line with the latest regulations.
Encryption and Access Control
One of the fundamental aspects of data security is encryption, which involves converting data into a code to prevent unauthorized access. AI algorithms can help in creating more robust encryption techniques. Similarly, AI can manage role-based access control systems more efficiently, ensuring that only authorized personnel have access to sensitive data.
Challenges and Future Directions
While AI offers promising solutions, it also presents challenges. The algorithms themselves need to be secure to prevent manipulation. Also, as AI systems require access to large datasets for training, ensuring the privacy and security of this data is crucial. Looking ahead, the integration of blockchain technology and AI could offer a more secure and transparent way to manage healthcare data.
Section 2: AI Algorithms and Their Applications
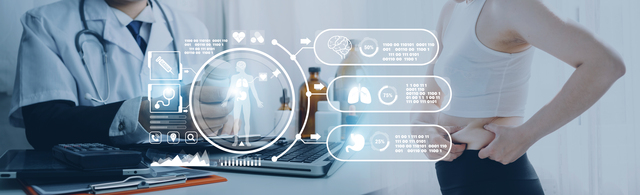
Machine Learning Models: Supervised, Unsupervised, and Reinforcement Learning
Machine learning models in healthcare come in various forms, each with its unique advantages and applications. Supervised learning algorithms are often used for diagnostic purposes, where labeled data is available. Unsupervised learning can be used for clustering patient data and identifying hidden patterns. Reinforcement learning, although less commonly used, has potential in personalized treatment plans where the algorithm learns the best course of action through trial and error.
Natural Language Processing for Medical Records
Natural Language Processing (NLP) is becoming increasingly important in healthcare for the analysis and interpretation of medical records. By automating the extraction of valuable information from unstructured text, NLP can significantly reduce the administrative burden on healthcare providers and improve the quality of care.
Computer Vision for Medical Imaging
Computer vision algorithms are revolutionizing medical imaging by providing more accurate and faster diagnoses. These algorithms can detect abnormalities in X-rays, MRIs, and CT scans with high precision, aiding radiologists and reducing human error.
Sub-section: Predictive Analytics
Predictive Models for Patient Outcomes
Predictive analytics in healthcare can forecast various patient outcomes, such as readmission risks, potential complications, and even patient mortality. These models use historical and real-time data to make these predictions, allowing healthcare providers to take preventive measures.
AI in Drug Discovery and Genomics
AI algorithms are speeding up the drug discovery process and are making significant contributions to genomics. They can analyze complex biochemical interactions and can even predict how a drug will interact with targets in the body.
Real-time Analytics for Critical Care
Real-time analytics are crucial in critical care settings where timely decisions can be the difference between life and death. AI algorithms can continuously monitor patient vitals and other critical data to alert healthcare providers of any sudden changes that require immediate attention.
Also read – Predictive AI: Foreseeing the Future
Section 3: Cloud Automation and Migration
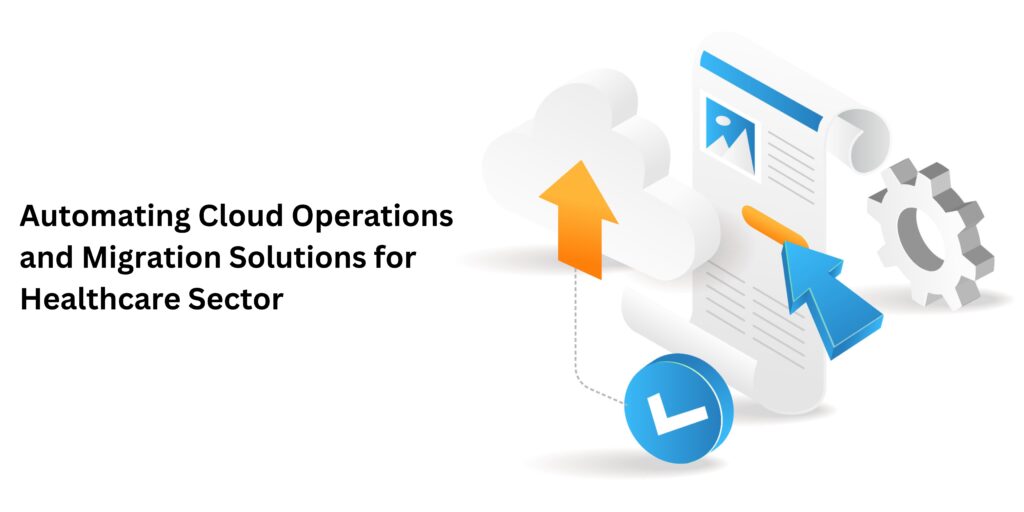
Importance of Cloud Computing in Healthcare
Cloud computing offers scalable and flexible solutions that are crucial for data-intensive tasks in healthcare. It provides a secure and efficient way to store and manage vast amounts of patient data and supports advanced analytics and machine learning algorithms.
Strategies for Effective Cloud Migration
Migrating to the cloud is not a straightforward task and requires a well-thought-out strategy. This includes choosing the right cloud service model, ensuring data security and compliance, and training the staff for the new environment.
Automation Tools and Their Benefits
Automation tools in cloud computing can handle repetitive tasks, reduce errors, and free up human resources for more complex tasks. They can automate data backups, software updates, and even some aspects of data analysis, making the healthcare processes more efficient.
Section 4: Data Consulting and Strategy
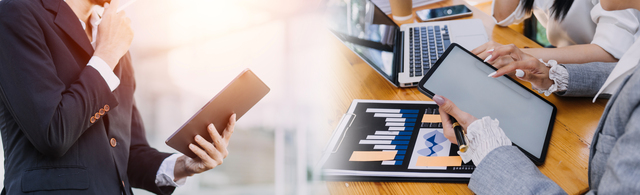
Role of Data Scientists and Analysts
Data scientists and analysts play a crucial role in healthcare analytics. They not only prepare and analyze data but also interpret the results to provide actionable insights. Their work forms the backbone of any data-driven initiative in healthcare.
Data-Driven Decision-Making
Data-driven decision-making involves using statistical tools and algorithms to make decisions based on data rather than intuition or observation alone. In healthcare, this means using data to decide on treatment plans, allocate resources, and even predict patient outcomes.
KPIs and Metrics for Healthcare Analytics
Key Performance Indicators (KPIs) and metrics provide measurable values that demonstrate the effectiveness of a healthcare organization in achieving its objectives. These can include patient satisfaction rates, readmission rates, and the success rates of treatments, among others. These metrics are crucial for assessing the performance and making necessary adjustments for improvement.
Section 5: Case Studies
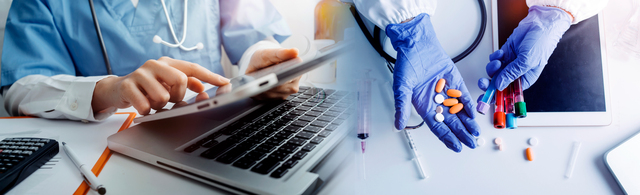
Implementing AI for Predictive Diagnostics
In a recent case study, a renowned healthcare institution successfully implemented AI algorithms for predictive diagnostics. The system could accurately predict the likelihood of various diseases, including diabetes and cardiovascular disorders, allowing for early interventions and better patient outcomes.
Data Security Measures in a Large Healthcare Provider
Another case study focuses on a large healthcare provider that implemented robust data security measures. They employed end-to-end encryption, multi-factor authentication, and regular security audits to protect patient data, achieving a significant reduction in data breaches.
Cloud Migration for a Multi-Facility Hospital
A multi-facility hospital recently migrated its entire data infrastructure to the cloud. The move not only streamlined operations but also provided a more secure and scalable environment. The hospital now enjoys reduced operational costs and improved data accessibility.
Section 6: Challenges and Ethical Considerations
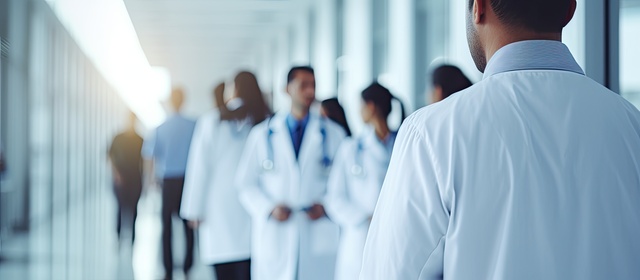
Data Bias and Fairness
One of the significant challenges in implementing AI in healthcare is data bias. If the data used to train AI models is biased, the predictions and recommendations will also be biased, leading to unfair and potentially harmful outcomes.
Ethical Considerations in AI Implementations
Ethical considerations are paramount when implementing AI in healthcare. Issues such as patient consent for data usage and the potential for job displacement due to automation need to be carefully considered.
Regulatory Challenges
Healthcare is a highly regulated industry, and implementing new technologies like AI and cloud computing comes with its set of regulatory challenges. Ensuring compliance with laws like HIPAA in the U.S. is crucial for any healthcare organization.
Section 7: Future Trends
AI in Telemedicine
The future of healthcare is likely to see a significant rise in telemedicine, facilitated by AI algorithms. These algorithms can assist in remote diagnostics and monitoring, making healthcare more accessible.
Blockchain for Data Security
Blockchain technology has the potential to revolutionize data security in healthcare. By creating a secure and immutable ledger of transactions, blockchain can significantly reduce the risk of data breaches.
Quantum Computing in Healthcare
Quantum computing holds the promise of solving complex problems in seconds, which would take traditional computers years to solve. Its potential applications in healthcare include drug discovery and complex genetic research.
Conclusion
Summary of Key Points
This article has covered the transformative potential of Data and AI in healthcare, from predictive diagnostics to data security and cloud migration.