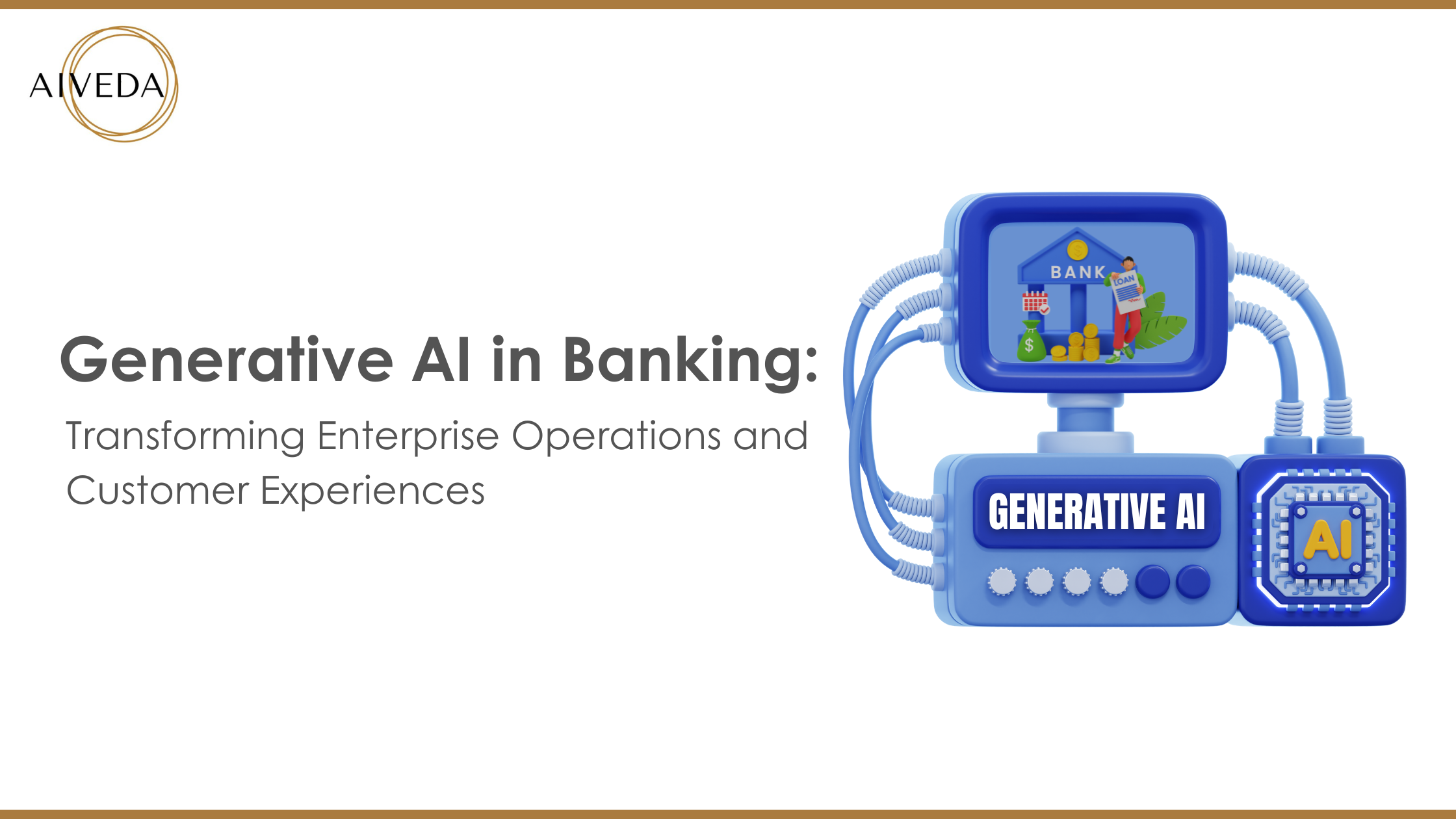
Introduction: Revolutionizing Banking with Generative AI
In today’s rapidly evolving financial landscape, banks and financial institutions are continually seeking innovative solutions to stay competitive and meet the dynamic needs of their customers. Generative AI (Gen AI) has emerged as a transformative technology, offering unprecedented opportunities to enhance operations, improve customer experiences, and drive strategic growth. This comprehensive guide delves into the profound impact of Generative AI in banking, exploring its multiple use cases, implementation strategies, and the tangible benefits it brings to enterprise banking solutions.
Section 1: Understanding Generative AI in Banking
What is Generative AI in Banking?
Generative AI refers to a subset of artificial intelligence that can create new content, such as text, images, or data, by learning patterns from existing information. In the banking sector, Generative AI leverages advanced machine learning algorithms to analyze vast amounts of financial data, enabling banks to generate insights, automate processes, and offer personalized services. Unlike traditional AI models that focus on predictive analytics, Generative AI can simulate human-like understanding and creativity, making it a powerful tool for innovation in banking.
Core Technologies Behind Generative AI
At the heart of Generative AI are several key technologies:
- Machine Learning (ML): ML algorithms enable systems to learn from data and improve over time without explicit programming.
- Deep Learning: A subset of ML, deep learning uses neural networks with multiple layers to model complex patterns in data.
- Natural Language Processing (NLP): NLP allows machines to understand, interpret, and generate human language, facilitating seamless communication between banks and customers.
- Computer Vision: This technology enables the analysis and interpretation of visual data, useful in areas like fraud detection and document processing.
The Role of Gen AI in Financial Services
Generative AI is reshaping financial services by enhancing data analysis, automating complex workflows, and enabling more informed decision-making. Banks utilize Gen AI to streamline operations, reduce costs, and deliver superior customer experiences. Gen AI is an indispensable asset in modern banking, from personalized banking solutions to advanced fraud detection mechanisms.
Section 2: The Strategic Importance of Gen AI in Banking
Current Challenges in the Banking Industry
The banking sector faces several challenges that hinder growth and efficiency:
- Increasing Competition: With the rise of fintech startups and digital banks, traditional banks are under pressure to innovate and differentiate their services.
- Regulatory Compliance: Navigating complex regulatory requirements demands robust systems to ensure adherence and mitigate risks.
- Operational Inefficiencies: Legacy systems and manual processes can lead to inefficiencies, higher operational costs, and slower service delivery.
- Customer Expectations: Today’s customers demand seamless, personalized, and instant banking experiences, pushing banks to adopt advanced technologies.
How Generative AI Addresses These Challenges
Generative AI offers targeted solutions to these challenges:
- Enhancing Operational Efficiency: By automating routine tasks and optimizing workflows, Gen AI reduces operational costs and improves service delivery.
- Improving Customer Engagement: AI-driven personalization ensures that customers receive tailored financial products and services, enhancing satisfaction and loyalty.
- Strengthening Security and Fraud Detection: Advanced AI models can detect unusual patterns and potential fraud in real-time, safeguarding both the bank and its customers.
- Ensuring Regulatory Compliance: Gen AI can automate compliance checks and generate accurate reports, ensuring adherence to regulatory standards.
Section 3: Key Use Cases of Generative AI in Banking
1. Fraud Detection and Prevention
Generative AI revolutionizes fraud detection by analyzing vast datasets to identify suspicious activities. Real-time anomaly detection algorithms can flag unusual transactions, enabling banks to respond promptly and prevent potential fraud. Predictive analytics further enhance these capabilities by forecasting fraudulent behavior based on historical data patterns.
Case Study: A leading global bank implemented Gen AI for fraud detection, resulting in a 30% reduction in fraudulent transactions within the first year. The AI model’s ability to learn and adapt to new fraud patterns significantly enhanced the bank’s security measures.
2. Customer Service and Chatbots
AI-powered chatbots provide 24/7 customer support, handling inquiries ranging from account information to transaction queries. Utilizing Natural Language Processing (NLP), these chatbots understand and respond to customer needs effectively, improving satisfaction and reducing the burden on human support teams.
Example: A major bank deployed a Generative AI chatbot that handled over 60% of customer inquiries autonomously, leading to faster response times and increased customer satisfaction.
3. Personalized Banking and Marketing
Generative AI enables banks to offer personalized financial products and services based on individual customer profiles and behavior. By analyzing data such as transaction history and spending patterns, AI models can recommend tailored solutions, enhancing cross-selling and up-selling opportunities.
Impact: Personalized marketing campaigns driven by Gen AI have been shown to increase conversion rates by up to 25%, as customers receive relevant and timely offers aligned with their financial needs.
4. Risk Management and Compliance
Gen AI ensures that banks adhere to regulatory standards efficiently by automating compliance checks and risk assessments. Predictive risk modeling allows for better decision-making by forecasting potential risks and enabling proactive measures.
Implementation: Banks using Gen AI for compliance reporting have experienced a 40% reduction in manual processing time, allowing compliance teams to focus on strategic initiatives rather than routine checks.
5. Automated Document Processing
Generative AI streamlines document-intensive processes such as loan applications and Know Your Customer (KYC) procedures. Optical Character Recognition (OCR) combined with AI-driven data extraction minimizes manual errors and accelerates processing times.
Benefits: Automated document processing has led to a 50% decrease in loan application processing time, enhancing customer experience and operational efficiency.
6. Predictive Analytics for Investment
AI-driven investment strategies and portfolio management utilize Gen AI to analyze market trends and forecast investment opportunities. This enhances the accuracy of investment decisions and optimizes portfolio performance.
Result: Banks employing predictive analytics with Gen AI have seen a 15% improvement in investment returns, driven by more informed and timely decision-making.
7. Loan and Credit Assessment
Generative AI automates credit scoring and risk assessment, providing a more accurate evaluation of loan applicants. This reduces default rates by identifying high-risk individuals and streamlining the approval process.
Example: An enterprise bank integrated Gen AI into its credit assessment system, resulting in a 20% reduction in loan default rates and faster approval times.
Section 4: Implementing Generative AI in Banking Enterprises
1. Strategic Planning and Roadmap Development
Successful Gen AI implementation begins with strategic planning. Enterprises must define clear objectives and key performance indicators (KPIs) to align AI initiatives with business goals. Developing a comprehensive roadmap ensures a structured approach to integrating Gen AI technologies.
Steps:
- Define Objectives: Identify specific areas where Gen AI can add value, such as customer service or risk management.
- Set KPIs: Establish measurable outcomes to track the success of AI initiatives.
- Develop a Roadmap: Create a timeline with milestones for AI integration and deployment.
2. Data Management and Preparation
Data is the backbone of Generative AI. Ensuring data quality and integrity is crucial for accurate AI modeling. Banks must implement robust data governance frameworks to manage data securely and comply with regulatory requirements.
Best Practices:
- Data Cleaning: Remove inaccuracies and inconsistencies from datasets.
- Data Integration: Consolidate data from various sources for a comprehensive view.
- Data Security: Implement encryption and access controls to protect sensitive information.
3. Technology Stack and Tools
Selecting the right technology stack is essential for effective Gen AI implementation. Banks should choose platforms and frameworks that support scalability, integration, and advanced AI capabilities.
Components:
- AI Platforms: Solutions like TensorFlow, PyTorch, and IBM Watson offer robust AI functionalities.
- Integration Tools: APIs and middleware facilitate seamless integration with existing banking systems.
- Data Storage: Cloud-based storage solutions provide the scalability needed for large datasets.
4. Building and Training AI Models
Developing effective AI models requires a combination of expertise and the right tools. Banks should leverage best practices for model development, including the use of transfer learning and pre-trained models to accelerate the training process.
Steps:
- Model Selection: Choose appropriate algorithms based on the use case.
- Training Data: Use diverse and representative datasets to train models.
- Validation: Continuously test and refine models to ensure accuracy and reliability.
5. Deployment and Scalability
Deploying Gen AI solutions at scale involves ensuring that AI models perform reliably in production environments. Banks must implement strategies for seamless deployment and scalability to handle varying workloads and traffic volumes.
Strategies:
- Containerization: Use Docker and Kubernetes for scalable deployments.
- Monitoring: Implement real-time monitoring to track performance and identify issues.
- Load Balancing: Distribute workloads evenly to prevent bottlenecks.
6. Monitoring and Continuous Improvement
Continuous monitoring and iterative improvements are essential for maintaining the effectiveness of Gen AI solutions. Banks should use performance tracking tools to monitor AI models and make data-driven adjustments as needed.
Tools:
- Monitoring Tools: Solutions like Prometheus and Grafana provide real-time insights into AI performance.
- Feedback Loops: Collect feedback from stakeholders to inform model enhancements.
- Regular Updates: Keep AI models updated with the latest data and algorithms to maintain accuracy.
Also read: Generative AI in BFSI
Section 5: Benefits of Generative AI in Banking
1. Operational Efficiency and Cost Reduction
Generative AI automates repetitive tasks, reducing the need for manual intervention and lowering operational costs. By streamlining workflows, banks can achieve higher productivity and allocate resources more effectively.
Impact:
- Cost Savings: Automation can lead to significant cost reductions by minimizing labor-intensive processes.
- Efficiency Gains: Faster processing times enhance overall operational efficiency, allowing banks to serve more customers efficiently.
2. Enhanced Customer Experience
Personalized and seamless banking experiences are now achievable through Gen AI. By tailoring services to individual needs, banks can improve customer satisfaction and foster loyalty.
Benefits:
- Personalization: AI-driven insights enable the customization of financial products and services.
- Seamless Interactions: Enhanced user interfaces and AI-powered support ensure smooth and efficient customer interactions.
3. Improved Decision-Making
Data-driven insights provided by Gen AI support more informed and strategic decision-making. By analyzing vast amounts of data, AI models help banks identify trends, predict outcomes, and make proactive decisions.
Advantages:
- Strategic Insights: AI-generated analytics provide deep insights into market trends and customer behavior.
- Reduced Bias: Automated decision-making processes minimize human bias, leading to more objective outcomes.
4. Competitive Advantage
Adopting Generative AI gives banks a significant edge over competitors. By leveraging innovative AI-driven solutions, banks can differentiate their services and stay ahead in a crowded market.
Outcomes:
- Innovation Leadership: Banks that embrace Gen AI are seen as leaders in innovation, attracting more customers and partners.
- Market Differentiation: Unique AI-powered services set banks apart from traditional competitors, driving growth and market share.
Section 6: Challenges and Considerations in Gen AI Adoption
1. Data Privacy and Security
Protecting sensitive customer data is paramount in banking. Implementing robust security measures and ensuring compliance with data protection regulations are critical when adopting Gen AI.
Challenges:
- Regulatory Compliance: Navigating complex data protection laws such as GDPR and CCPA.
- Data Security: Safeguarding data against breaches and unauthorized access.
2. Ethical Considerations
Addressing ethical concerns is essential to maintain trust and fairness in AI-driven processes. Banks must ensure that their AI models are free from bias and operate transparently.
Concerns:
- Bias and Fairness: Ensuring AI models do not perpetuate existing biases in data.
- Transparency: Providing clear explanations for AI-driven decisions to stakeholders.
3. Integration with Legacy Systems
Many banks rely on legacy systems that may not be compatible with modern AI technologies. Overcoming technical debt and ensuring seamless integration is a significant challenge.
Solutions:
- API Integration: Using APIs to bridge the gap between old and new systems.
- Modular Architecture: Adopting a modular approach to facilitate easier integration and scalability.
4. Skill Gaps and Training
Implementing Gen AI requires specialized skills that may be scarce within the existing workforce. Banks must invest in training and hiring to build the necessary expertise.
Strategies:
- Training Programs: Developing internal training initiatives to upskill employees.
- Hiring Experts: Bringing in external talent with specialized AI knowledge.
5. Regulatory Compliance
Staying compliant with evolving regulations around AI use in banking is crucial. Banks must implement robust compliance frameworks to navigate the regulatory landscape effectively.
Approaches:
- Compliance Frameworks: Establishing comprehensive frameworks to guide AI implementation.
- Regular Audits: Conducting regular audits to ensure ongoing compliance with regulatory standards.
Section 7: Future Trends of Generative AI in Banking
1. Advancements in AI Technologies
The field of AI is continuously evolving, with new techniques and technologies emerging. Future advancements in Gen AI will further enhance its capabilities and applications in banking.
Trends:
- Quantum Computing: Leveraging quantum computing to solve complex AI problems more efficiently.
- Advanced Neural Networks: Developing more sophisticated neural networks for deeper insights and predictions.
2. AI-Driven Financial Products and Services
Generative AI will drive the creation of innovative financial products and services, offering customers unprecedented levels of customization and functionality.
Examples:
- AI-Enabled Advisory Services: Providing personalized financial advice based on individual customer data.
- Smart Contracts: Utilizing AI to automate and enforce contractual agreements seamlessly.
3. Sustainable and Responsible AI
Integrating sustainability goals with AI initiatives will become increasingly important. Banks will focus on using AI responsibly to promote ethical practices and sustainable growth.
Initiatives:
- Green AI: Developing AI solutions that are energy-efficient and environmentally friendly.
- Ethical AI Practices: Ensuring AI models are designed and used in ways that promote fairness and transparency.
4. Collaborations and Ecosystem Development
Collaborations between banks, technology providers, and AI experts will foster innovation and accelerate the adoption of Gen AI in the financial sector.
Collaborative Efforts:
- Partnerships with AI Firms: Working with specialized AI companies to develop tailored solutions.
- AI Ecosystems: Building interconnected ecosystems that facilitate shared innovation and growth.
Section 8: Real-World Case Studies
Case Study 1: Gen AI for Enhanced Fraud Detection
Background and Objectives:
A leading multinational bank sought to enhance its fraud detection capabilities to protect its customers and assets better. The primary objective was to reduce fraudulent transactions and improve the overall security framework.
Implementation Process and Technologies Used:
The bank integrated Gen AI models with its existing transaction monitoring systems. Utilizing machine learning algorithms and real-time data analysis, the AI system could identify unusual patterns indicative of fraudulent activities.
Outcomes and Measurable Benefits:
Post-implementation, the bank saw a 30% reduction in fraudulent transactions. The real-time detection capabilities enabled quicker responses, minimizing potential losses and enhancing customer trust.
Case Study 2: AI-Powered Personalized Banking Services
Background and Objectives:
A regional bank aimed to improve customer engagement by offering personalized financial products and services. The goal was to increase customer satisfaction and loyalty through tailored banking experiences.
Implementation Process and Technologies Used:
The bank deployed Generative AI to analyze customer data, including transaction history and spending patterns. The AI models generated personalized product recommendations and targeted marketing campaigns based on individual customer profiles.
Outcomes and Measurable Benefits:
The personalized approach led to a 25% increase in product adoption rates and a 20% boost in customer retention. Customers appreciated the tailored services, which significantly enhanced their banking experience.
Case Study 3: Automating Compliance with Gen AI
Background and Objectives:
A major financial institution needed to streamline its compliance processes to adhere to stringent regulatory requirements. The objective was to reduce manual intervention and enhance the accuracy of compliance reporting.
Implementation Process and Technologies Used:
Generative AI was implemented to automate compliance checks and generate accurate reports. The AI system analyzed regulatory data and ensured that all transactions and operations met the necessary standards.
Outcomes and Measurable Benefits:
The automation of compliance processes resulted in a 40% reduction in manual processing time. The bank achieved higher accuracy in reporting, reducing the risk of non-compliance and associated penalties.
Conclusion: Embracing Generative AI for a Future-Ready Banking Enterprise
Generative AI is undeniably reshaping the banking industry, offering transformative solutions that enhance operational efficiency, improve customer experiences, and drive strategic growth. By leveraging Gen AI, banks can navigate the complexities of modern financial services, stay ahead of the competition, and deliver unparalleled value to their customers.
Recap of Gen AI’s Impact on Banking:
From fraud detection and personalized services to risk management and automated compliance, Generative AI addresses critical challenges and unlocks new opportunities for banks.
Strategic Imperative for Enterprises:
Adopting Gen AI is no longer optional but a strategic imperative for banks aiming to remain competitive and relevant in an increasingly digital world.